Laws & Theorem
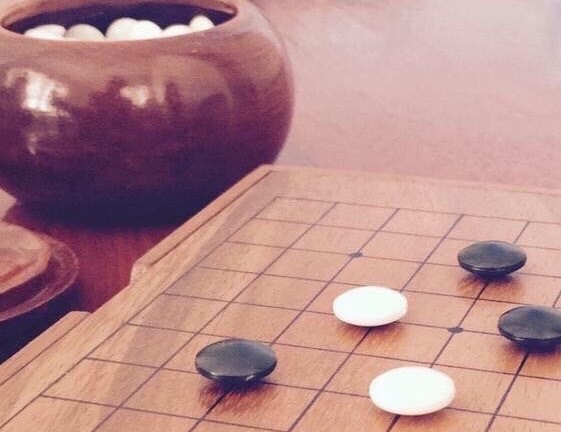
Transitive Laws
Let A and B be 9 × 9 Go bots with superhuman neural networks. If A topped the chart of a human Go server, then B can top that chart too.
Proof
KataTest 9 Dan (20b9_335 run on RTX 2060) topped the chart of Go Quest 9 x 9 Go server in Sep. 2019 (+94-0=1). Monteo da Westi, playing 102 games of 9 × 9 Go for A Master of Go, with KataGo engine and the old neural network (2019) topped the same chart in August 2020 (+100-1=1).
Insight
A Master of Go (v6.0.1), with the new KataGo engine and stronger neural network (Kata1_b18nbts550), can top the chart of Go Quest.
Performance Theorem
Let a proper komi for 9 × 9 Go be 7. Assume that the neural network has been trained to perfection. If komi is 7.5, then White's win rate equals 100% at Move 1. Besides, the weaker neural network, the lower win rate for White at Move 1.
Proof
We know that the currently strongest confidently-rated network is stronger than the previous ones. The neural networks can be arranged by strength as 170e_b10s114 < 170e_b20s530 < Kata1_b18nbts550, confirmed by the fact that the win rates for White at Move 1 of these neural networks were 62%, 85%, and 88%, respectively, according to A Master of Go 9p+.
Insight
The performace of the new naural network, Kata1_b18nbts550, is probably 88% of the maximum achievable performance. This theorem can be useful for checking how well a neural network has been trained, by showing the performance gap between the theoretically perfect neural network and the one being tested.